Framing stronger hypotheses with elaboration, iteration, and a Mad Lib
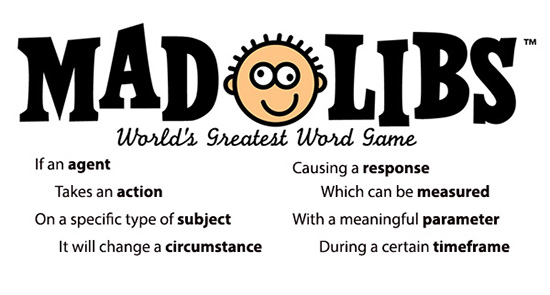
This idea was first taught at Rutgers University / and first published on ConnectiveDX.com
As part of my teaching at Rutgers Business School, I was recently leading an innovation workshop for a technology company. They are well-known inventors, and research and discovery are a big part of their culture. You can call it being fact-based, empirical, or scientific — the practice of testing and moving forward with a rational expectation for what happens next is a priority for leaders across disciplines.
But the insights we have grow from the hypotheses we frame. So, our workshop dug-in to what makes a hypothesis strong. You’ll find these ideas distilled in to a Mad Lib worksheet later in the post. But before that, I’d like to show why better hypotheses make testing more impactful.
Ideas start life as weak hypotheses
When someone has an idea, the impulse to quickly test and see what happens makes perfect sense. But, without further iterations, these “let’s see” tests may go forward simply as if-then statements. Consider this marketing hypothesis, “if we show ad Z, then people will buy more.” That sounds like a positive outcome. But if we proceed to test with nothing more than a weak hypothesis and a bias toward action, the resulting findings may tell us less than we imagine.
That’s because the hypothesis leaves a lot out. Why exactly would customers buy more? Is it because ad Z is somehow better than other ads, or is this just a demonstration that some promotion is better than none at all? If the later is true, we might best move on. Meaningless A/B tests are becoming pervasive because they are so easy to run, and they give an illusion of process and certainty without new insight.
Elaboration enables iteration and more impactful insights
Hypotheses get stronger through a process of elaboration which increases their specificity while making more of their assumptions explicit. Also, my colleagues and I have found that well-elaborated hypotheses are easier to connect with one another. This allows results from waves of iterative testing to advance more general concepts.
I had the chance to hear Roy Rosin, the Chief Innovation Officer at Penn Medicine’s Center for Healthcare Innovation outline the kind of questions he asks to help refine hypotheses.
- Can you predict the anticipated change more specifically?
- Why do you believe this will happen, what mechanism will deliver it?
- Who will be impacted, by when?
Building on his approach, my class and I assembled an “insert-the-word” Mad Lib to organize on the kinds of questions Rosin promotes asking.
- If an agent:
- Takes an action:
- On a specific type of subject:
- It will change a circumstance:
- Causing a response:
- Which can be measured:
- With a meaningful response in this parameter:
- During a certain timeframe:
Used this way, our Mad Lib is a conceptual checklist which encourages teams to reflect on their tests systematically. I’m curious if there are other dimensions you find important for framing investigations? Does this list seem applicable to hypotheses across disciplines and industries? By all means, please add your thoughts in the discussion section below.
Putting this technique to work
My class applied this rubric on a case we discussed based on an initiative led by Connective DX’s Director of Insights and Optimization, Ryan Summers. His team collaborated with a consumer service firm which took an experimental approach to website personalization. They investigated if they could infer what mattered most to certain kinds of buyers by watching their website behavior, and then if showing them messages addressing these interests might increase their intent to purchase. We applied the Mad Lib approach to review this test structure:
- If an agent: the content management system of their enterprise website.
- Takes an action: shows a personalized content and calls to action.
- On a specific type of subject: based on the visitor’s inferred priorities (mindset).
- It will change a circumstance: increase their wish to consider a purchase of services.
- Causing a response: a. increased search for local service points; or b. more requests for sales contact.
- Which can be measured: with data from their content management system or marketing automation system.
- With a meaningful response in this parameter: 10% more visits to local location pages and 5% more requests for contact.
- During a certain timeframe: in all of all website visits identified from that person over the next thirty days.
The test confirmed personalized content targeting drove positive outcomes. But, did this confirm that the “buyer mindsets” are actually on target? Not necessarily. It might suggest that any personalized message compares favorably with the default message seen by the control group. But iterative testing helped prove this out.
Iteration builds knowledge from results
The next tests again inferred visitors’ priorities, but this time the personalized content shown to them was randomly assigned. A cross-tabulation of these results confirmed most groups were best addressed with the messages written just for them. And further, the results also showed which alternative messages for other groups had a negative effect on each kind of visitor.
Since accurately reading the interests of anonymous website visitors was the core of this method, the team found a way to gauge how accurately they were doing this. Once the site inferred a visitor’s interest, they were surveyed to see if their self-reported priorities matched those predicted by the experience design team’s model.
These waves of iterative testing helped advance their thinking and develop a durable insight which could be useful across their organization. This is the opposite of one of those “Let’s see” tests. And while this needn’t be the standard for all testing, it’s a reminder of how impactful smart testing can be.
Even quick “Let’s see” testing can benefit from elaboration
Simply testing-in a great new idea to confirm that it works is still smart. But even these quick tests can benefit from even minimal elaboration. Here are two creative ideas people have shared with me recently:
- How might one measure the effect of those Best Hospital or Best College ranking logos so often seen on their homepages?
- Can the contact method on the website be switched from phone to other channels that are more operationally efficient without losing leads?
“Let’s see” is a good reply to align innovators and encourage decisions based on evidence rather than influential opinions. Adding a brief discussion to elaborate on these ideas can help tighten their focus, and align team members expectations.
- For testing rankings icons on websites: one could consider their effects on existing customers, first-time visitors or job seekers separately. While everyone wants to measure to admissions or inquiries, what about seeing if visitors remain engaged on these sites longer?
- For replacing phone contact with other methods: not all leads are equal, so would it make sense to measure their value beyond counting them? Do certain demographic groups respond differently to this shift? Would having a test state promoting all contact methods increase lead volume, or is the demand so inelastic so that changing contact method redistributes the same buyers?
Gaining More Business Impact from Testing
Developing meaningful ideas that can be implemented can be a long road. Few of these concepts may ever be prototyped or tested. So considering the best ways to measure and structure tests can increase their business impact through new customer insights. This Madlibs approach is a reminder that if you’re going to test ideas, you can get more value by formalizing and elaborating a strong hypothesis.
3 Responses to "Framing stronger hypotheses with elaboration, iteration, and a Mad Lib"
July 2, 2019
[…] If you’d like to dig-in to specifics, I’ve written about lessons from Penn Medicine on constructing better hypotheses for testing. […]
September 15, 2020
Relax yourself a little with free sexy chat at shemale gold coast! Hot ladyboys are waiting for you!
July 17, 2025
I was recently in charge of an innovation workshop for a technology company while I was teaching at Rutgers Business School.